In recent years, AI in insurance fraud detection has emerged as a transformative force within the industry. As fraud schemes become increasingly sophisticated, traditional methods of detection are proving insufficient. Artificial Intelligence (AI) offers innovative solutions that promise to enhance accuracy and efficiency in identifying fraudulent claims. However, integrating AI into fraud detection systems presents both groundbreaking opportunities and significant challenges. This article explores how AI is reshaping insurance fraud detection, highlighting the innovations driving change and the obstacles that need addressing.
Innovations in AI for Fraud Detection
AI technologies have revolutionized how insurance companies approach fraud detection. Machine learning algorithms, for instance, are capable of analyzing vast amounts of data at remarkable speeds. By identifying patterns and anomalies that might escape human analysts, these algorithms can flag potentially fraudulent claims with high precision. Predictive analytics further amplify this capability by forecasting future fraud trends based on historical data. For example, AI systems can detect emerging fraud patterns by examining trends and behaviors that indicate potential threats. The ability of AI to continuously learn and adapt to new data makes it an invaluable tool in combating ever-evolving fraudulent tactics.
In addition to pattern recognition, AI can utilize real-time data processing to enhance fraud detection. For instance, AI-powered systems can monitor transactions and claims as they are submitted, flagging suspicious activities immediately. This real-time capability allows insurers to act swiftly, reducing the potential for significant losses and improving overall fraud management.
Enhancing Accuracy with Advanced Algorithms
One of the key innovations in AI for fraud detection is the development of advanced algorithms that improve accuracy. Deep learning techniques, for example, enable AI systems to analyze complex data structures and identify subtle patterns indicative of fraud. These algorithms use neural networks that mimic human cognitive processes to evaluate claims with greater accuracy.
Natural language processing (NLP) is another significant advancement. NLP allows AI systems to analyze textual data from claim descriptions and communication between claimants and insurers. By detecting inconsistencies, deceptive language, or unusual patterns, NLP algorithms can identify potentially fraudulent claims that may not be evident through traditional methods. This level of scrutiny not only enhances accuracy but also reduces the incidence of false positives, ensuring that legitimate claims are processed more efficiently.
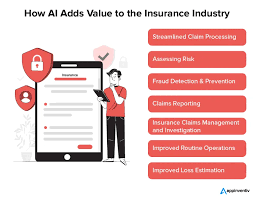
The Role of Data Integration
Effective AI fraud detection systems rely heavily on data integration. By consolidating information from various sources, including claims data, social media activity, and public records, AI systems can build a more comprehensive profile of each claim. This holistic view enhances the system’s ability to detect fraud by providing a broader context for analysis.
Data integration also facilitates advanced analytics. For instance, combining data from multiple sources allows AI systems to cross-reference information, verifying the accuracy of claims and identifying discrepancies. This integrated approach also supports real-time monitoring, enabling insurers to respond promptly to suspicious activities and mitigate potential losses.
Moreover, AI systems can leverage external data sources, such as geolocation data and historical claim trends, to improve fraud detection. By incorporating diverse data types, AI can offer a more nuanced understanding of each claim, enhancing the accuracy of fraud detection efforts.
Challenges in Implementing AI Solutions
Despite the promising advancements, implementing AI in insurance fraud detection comes with its own set of challenges. One significant issue is the quality of data. AI systems are only as effective as the data they are trained on. Inaccurate or incomplete data can lead to incorrect predictions and potentially miss fraudulent activities. Ensuring data quality requires ongoing efforts in data cleansing, validation, and enrichment.
Additionally, the complexity of AI algorithms can make them difficult to interpret, raising concerns about transparency and accountability. Insurers must ensure that AI systems are not only effective but also understandable and explainable. This is particularly important for regulatory compliance and maintaining trust with customers.
Addressing Ethical and Privacy Concerns
Ethical and privacy concerns also pose challenges in the deployment of AI for fraud detection. The use of AI involves processing large amounts of personal data, which can raise issues related to data protection and privacy. Insurers must ensure that their AI systems comply with regulations such as the General Data Protection Regulation (GDPR) and maintain strict data security protocols.
Balancing the need for effective fraud detection with the rights of individuals is a critical consideration. Insurers must implement robust privacy measures to protect sensitive information while leveraging AI to enhance fraud detection capabilities. This includes anonymizing data where possible and ensuring that AI systems do not inadvertently perpetuate biases or discrimination.
Future Directions for AI in Fraud Detection
Looking ahead, the future of AI in insurance fraud detection is promising. Ongoing advancements in technology are likely to lead to even more sophisticated algorithms and data integration techniques. For example, the integration of AI with blockchain technology could further enhance fraud detection by providing immutable and transparent records of transactions and claims.
Collaboration between insurers and technology providers will be essential in addressing current challenges and refining AI solutions. As AI continues to evolve, its potential to enhance fraud detection and contribute to more secure and efficient insurance processes will only grow. Future developments may include more advanced predictive models, improved real-time monitoring capabilities, and greater integration with external data sources.
In conclusion, AI is reshaping the landscape of insurance fraud detection with its innovative technologies and advanced algorithms. While challenges remain, the potential benefits of AI in improving accuracy and efficiency make it a crucial tool for combating fraud. By addressing these challenges and embracing future advancements, the insurance industry can leverage AI to create more robust and effective fraud detection systems.